Main Second Level Navigation
Breadcrumbs
- Home
- About
- News & Events
- News
- Medical Genomics Faculty Spotlight: Dr. Philip M. Kim
Medical Genomics Faculty Spotlight: Dr. Philip M. Kim
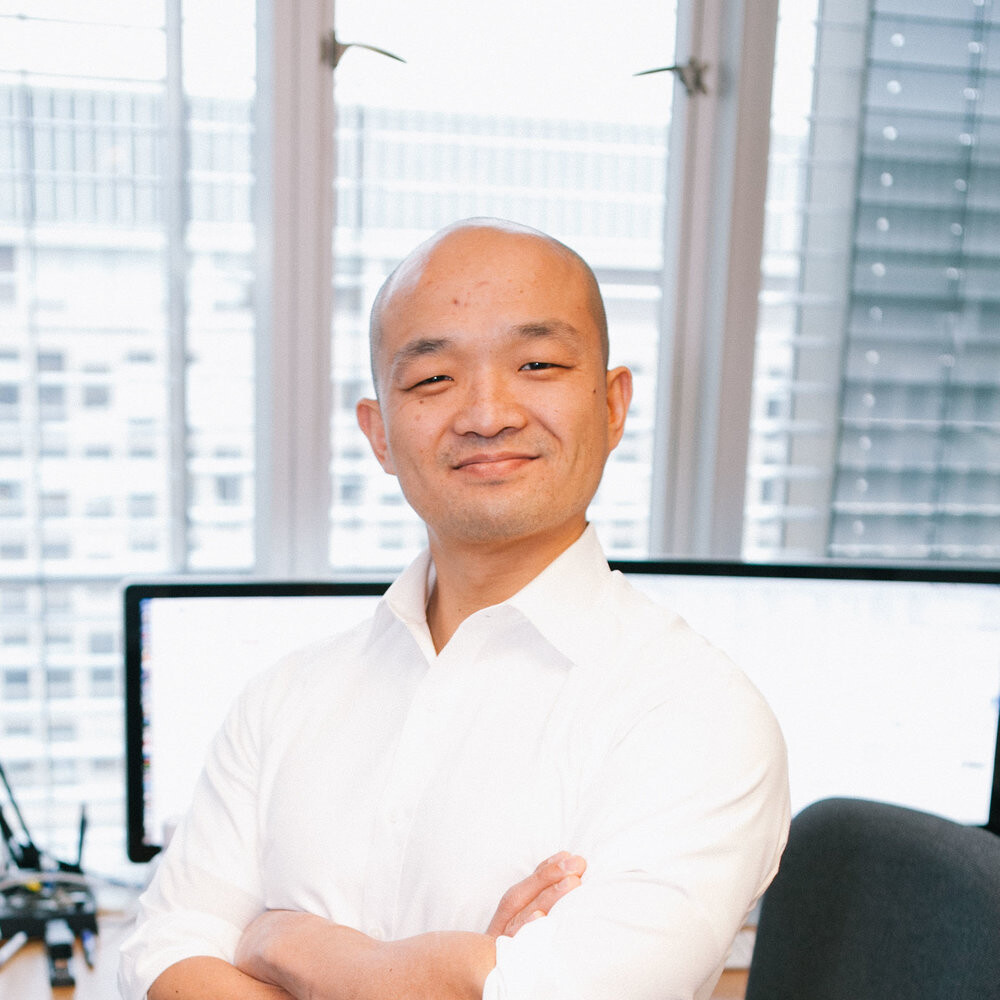
Dr. Philip M. Kim is a specialist in machine learning and computational biology. His current focus is on applying artificial intelligence (AI) to aid in the space of drug development. His paperrecently published in Nature Biotechnology in January of 2023 describes a new and comprehensive screening method for reprogramming zinc finger transcription factors (TFs) – protein molecules found in the nucleus of our cells, which interact with our DNA through direct physical contact. Dr. Kim’s research has already pushed the fields of protein and peptide design into uncharted territory, and the goal of his most recent work is now to accelerate the development of gene therapies.
Philip moved to Toronto about 14 years ago to conduct research at the University of Toronto and has been working in the broad area of biologics – protein-based therapeutics – ever since. In the Kim laboratory, machine learning algorithms capable of ‘deep learning’ are developed which integrate modern computational and experimental methodologies to solve problems in biomedical science. Philip did his BSc in Physics and Biochemistry at the University of Tübingen (in Germany, where he grew up), before moving to the United States to attend graduate school at MIT. He did his PhD there in Computational Biology and AI, with a specific focus in computational physical biochemistry. He performed a two-year stint with a large consulting group called McKinsey & Company, before becoming an associate research scientist and postdoctoral fellow at Yale University, for the next four years. Now based out of UofT, Philip’s research focuses on the design and validation of high-throughput computational methods to develop new protein-based therapeutics and develop new techniques for systems biology and translational science – two subspecialties of biology. Philip notes that he is “involved in a number of commercial ventures, mostly [focusing] around applications of a particular technology that [they] develop in the lab, and that can then be used to either directly develop drugs or help [research scientists] develop therapeutics.” The Kim lab develops “a whole range of techniques around the general theme of protein engineering and protein design.” Specifically, Dr. Kim designs therapeutics such as antibodies, medicinal peptides, novel gene therapies, and viral vectors engineered for delivery of these gene therapies. He principally does this through machine learning-based methods.
In conversation with Philip, he explained the differences between classical modelling (based in approximations to our classical understanding of physics) and deep learning (based on unbiased pattern recognition performed on real-world data): “If you took a protein structure, then classical modeling is based on approximations to the reality which is governed by quantum mechanics – and so [classical models] will always be approximations.” Philip continued, “There is a decent argument to be made that given enough data – and instead of a model based in classical physics – you can use a fairly complicated but fully unbiased model with hundreds of thousands, or millions of parameters […] to deduce protein structure.” In his opinion, protein structure deduction is “lowest hanging fruit” for deep learning-based applications in biology. In the Kim lab, they have seen substantial advances over the past couple of years in the ability to computationally model protein structure and design these protein-based therapeutics called ‘biologics.’
Computational (also known as in silico) drug design is near the beginning of the drug development pipeline. Once a protein-based drug candidate has been identified and validated in silico, the next step is laboratory-based wet lab work in molecular biology. Biologics designed in the Kim lab are thus like blueprints (or a recipe) for synthesizing these peptide-based therapeutics biochemically in the lab. Before it can ever be tested on a human in clinical trial, the lab-synthesized biologic must be validated as safe and effective from experiments performed on animal models. From this pre-clinical trial phase to the subsequent three clinical trial stages to FDA/Health Canada clearance, the drug development pipeline is long and rigorous, often taking many years and involving countless validation steps.
The Kim lab was one of the first in the world to harness machine learning algorithms for protein structure deduction and in silico biologic design: “We were among the very first to do actual machine learning for protein design and protein structure, and that goes back almost ten years now, and so we did some of the early work in [this space].” Dr. Philip Kim was recently recognized alongside post-doctoral fellow Dr. Pedro Valiente for creating mirror-image peptides that can neutralize SARS-CoV-2, the virus that causes COVID-19. Their paper published in the Journal of Medicinal Chemistry details how their new peptide technology was able to neutralize the virus and several of its variants and stop infection of cultured human cells. Since these mirror-image peptides are relatively cheap to produce and stable to degradation over time, the hope is that they can be active ingredients in low-cost antiviral therapeutics against SARS-CoV-2, potentially formulated for delivery as a nasal spray taken prophylactically. Because there are many validation steps required and regulatory hurdles to overcome before a drug can be administered to patients, these mirror-image peptide are likely still (at least) a couple years away from reaching the clinic.
Over the past ten years, the Kim lab has shifted gears from more traditional molecular biology benchtop science to applying machine learning to solve problems in biomedical science, principally in protein structure modelling and protein engineering. His work today is in systems biology, which is interdisciplinary, employing computational and mathematical analysis to model complex biological systems. This expertise led to Dr. Kim’s recruitment as a course instructor in the M.H.Sc. in Medical Genomics program at UofT. He teaches core statistical concepts to first-year graduate students in the program that he feels “anyone in the field should have a good grasp of” through our Biological Statistics course (MMG3002Y).
We are thankful to have had the opportunity to catch up with Dr. Philip Kim to hear more about his exciting projects in machine-learning-based computational drug design and are especially honoured to have him as a faculty member of the M.H.Sc. in Medical Genomics program. We wish Philip all the best in the Kim lab and beyond!