Main Second Level Navigation
Breadcrumbs
- Home
- About
- News & Events
- News
- Using Machine Learning to Find Antifungal Gene Targets in Candida albicans
Using Machine Learning to Find Antifungal Gene Targets in Candida albicans
Researchers in the Cowen lab collaborated domestically and across the border to create a gene-predicting machine learning model
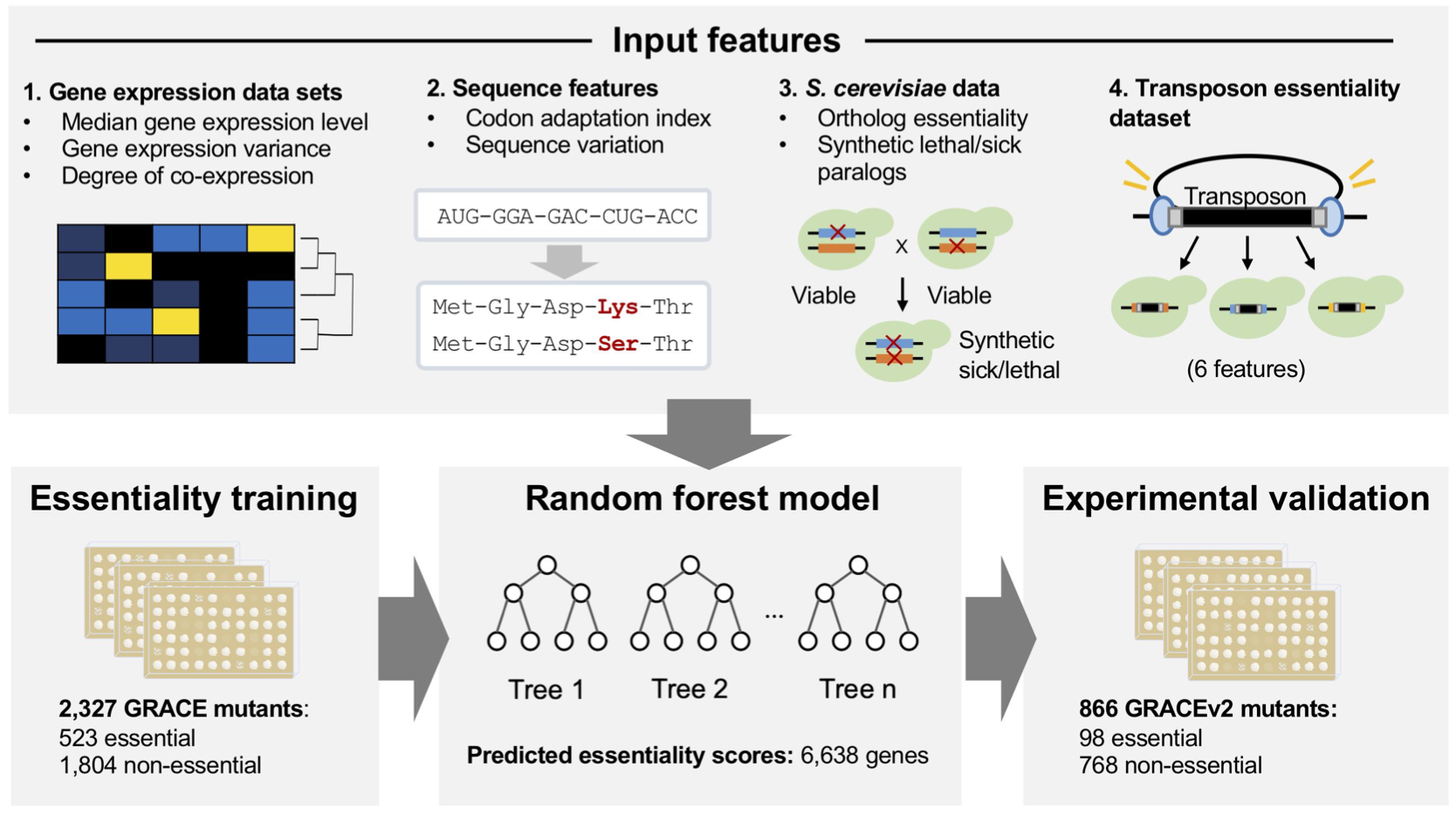
Although bacterial and viral pathogens have dominated the headlines in recent years, fungi are among these emerging microbial threats, especially those with suppressed or dysfunctional immune systems such as transplant recipients and cancer patients. Among these fungal pathogens are the Candida species, the leading cause of fungal infections in North America with a mortality rate of around 40% and a growing number of drug-resistant strains. There are limited numbers of antifungal options, with only three drug classes available, making the need for new therapeutics all the more essential. Genes found only in fungi, not found in humans, and necessary for fungal survival provide promising targets for new drugs. This promoted further research focused on genetic and genomic analyses for genes essential for fungal survival. One of the outcomes of said research was expanding the gene replacement and conditional expression (GRACE) collection, a mutant library that defines gene function in Candida albicans (C. albicans), the main disease-causing Candida species.
In the latest publication out of MoGen professor Dr. Leah Cowen’s lab, first authors Ci Fu, Amanda Veri, Kali Iyer, Emma Lash, Alice Xue and others built a machine learning model based on a type of algorithm called random forests (RF) to predict whether specific C. albicans genes are essential or not. Based on the model predictions, 866 additional mutants for the GRACE catalogue were generated, identifying 149 fungal-specific essential genes and discovering three previously unidentified genes—KRP1, EMF1, and TIF33—and their functions. The team also found a critical antifungal target in the form of the GLN4 gene.
The team developed and trained the machine-learning model to classify C. albicans genes as essential or not. They utilized multiple databases such as the GRACE library and other mutant collection datasets to collect information relevant to determining each gene’s essentiality and inserted said data into the machine-learning program. Then, the developers fed the program a set of known genes that have necessary functions. After integrating all this information, the program will train the machine-learning model to match the relevant information needed to determine if a gene is required or not with known features associated with essentiality, allowing the authors to make predictions for the rest of the fungal genes. The model managed to predict whether most C. albicans genes were essential, including 745 genes whose essentiality was previously unknown.
Subsequently, the team experimentally tested over 800 genes to see whether they were genuinely essential and found the prediction model to be highly accurate at a rate of 66% compared to previous methods, which were typically 36-47%. During this process, the team created a corresponding mutant fungal strain for each gene to observe the impact on growth, which allowed for expanded coverage of the C. albicans genome from 35% to 48%. The authors attribute this improved prediction to integrating several data sources and using individual features in the model. In addition, the authors experimentally confirmed 621 essential genes, including 53 that were newly identified and 149 that didn’t have human homologs/counterparts and were fungal-specific. The latter was significant since, as one of the first authors, Veri states: “Fungi are closely related to humans, so we need to be careful that the genes we target aren’t essential to humans as well.” They also conducted co-expression analyses, which analyzed and grouped genes with similar expression levels to determine biological processes/functions. First author Lash stated that co-expression analyses help with figuring out the role of genes with unknown purposes.
The research team then aimed to characterize the functions of three fungal-specific essential genes since they lack orthologs/counterparts in baker’s yeast and don’t have a known role. The first gene they characterized was KRP1, and it is a member of the kinetochore complex, which is involved in genome duplication during cell division. The second unidentified gene was named EMF1, implied to be a protein that binds to mitochondrial DNA based on co-expression analysis and its decreased expression leading to deformed mitochondria shape and reduced expression of mitochondrial genes. The third final gene was TIF33, determined to likely be a part of the eIF3 complex, which is responsible for initiating translation or protein production.
Additionally, the authors discovered that targeting the GLN4 gene provides a promising route for future drug targets. This gene encodes a glutaminyl-tRNA synthetase, an enzyme that attaches amino acids (the building blocks of proteins) to transfer RNAs (tRNAs), which carry the amino acids to the protein-making machinery of the cell. Indeed, mice infected with fungal strains with reduced Gln4 production didn’t succumb to illness, showcasing the enzyme’s importance for C. albicans spreading in its host. The authors also identified an antifungal compound that targets GLN4 called N- pyrimidinyl-beta-thiophenylacrylamide (NP-BTA), which they found to bind to Gln4 and inhibit its activity by preventing amino acids from docking. This molecule was able to block fungal growth in culture, further proving GLN4 as essential for the infectious ability of C. albicans.
For future directions, many exciting opportunities are now open. First author Fu mentioned that there is still a long list of unidentified and uncharacterized C. albicans genes that need to be studied. He elaborates further by highlighting that the team studied the genes under lab conditions, and they still need to be investigated in host and other environmental conditions to more accurately reflect real-world conditions of fungal infection. Furthermore, first author Xue noted that now that we have this database, we need to figure out the best strategy to discover genes to investigate in the future as some genes will be more important than others when it comes to leveraging the data for further drug discovery. She also mentions how this demonstrates a rare reversal in drug development, where the target is discovered before the compound itself.
Overall, this research illustrates why machine learning holds so much promise in the research sector, especially in genetics and antimicrobials. This methodology broadly applies to other pathogenic microbes and to figuring out unknown gene functions in other organisms. First author Fu notes future researchers can build how similar models with enough data to predict essential genes for other species. Lastly, it exemplifies the importance of collaboration and teamwork between labs domestically and internationally, as all the first authors clearly emphasized. This study involved the Cowen lab and another MoGen professor, Anne-Claude Gingras, and several labs from the United States, including the University of Minnesota, Minneapolis and the University of Michigan, Ann Arbor. “Expanding the coverage of the genome took two to three years and required concerted and collective effort from a great team,” noted first author Veri. “Collaboration both in and out of UofT and Canada was a special and memorable part of the project,” affirmed first author Lash, “it created an impactful paper.”
*Read the article here: Fu, C., Zhang, X., Veri, A.O. et al. Leveraging machine learning essentiality predictions and chemogenomic interactions to identify antifungal targets. Nat Commun 12, 6497 (2021).
*Check out first author Emma Lash’s Behind the Paper feature on Nature Portfolio Microbiology